Medical Imaging Informatics
|
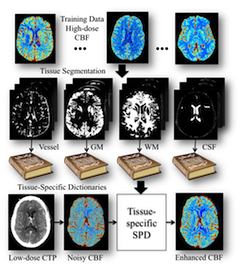 |
Tissue-Specific Sparse Deconvolution for Low-Dose CT Perfusion
We introduce a new robust deconvolution algorithm, using tissue-specific dictionaries in the learning and reconstruction, for low-dose CT perfusion. Benefited from the sparsity technique and tissue-discriminative nature, this algorithm is able to preserve the subtle perfusion information in the low-contrast tissue classes and vascular structure with improved performance. This is an extension of the MedIA'13 work on sparse perfusion deconvolution. It is applied to CT perfusion imaging of acute stroke patients.
-
Tissue-Specific Sparse Deconvolution for Low-Dose CT Perfusion.
[PDF]
[Project]
Ruogu Fang*, Tsuhan Chen, Pina Sanelli.
MICCAI, 2013. * Corresponding author
|
|
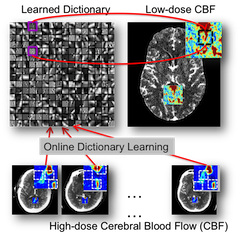 |
Robust Deconvolution via Sparse Perfusion Deconvolution
This project is a collaboration with
Weill Cornell Medical College (New York, NY.). We propose a novel sparse perfusion deconvolution method to perform robust perfusion parameter estimation, especially in low-dose, noisy image data. The method learns from the high-dose, high-quality perfusion maps and build a compact dictionary for robust deconvolution. The method can robustly handle outliers, avoid over-estimation of cerebral blood flow and preserve structural details. It is successfully applied to low-dose CT perfusion data of aneurysmal subarachnoid hemorrhage patients for ischemic voxles detection.
|
|
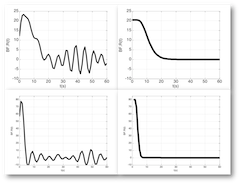 |
Sparse Residue Function Recovery in Low-Dose CTP Imaging
We use the high-dose residue function in 4D spatio-temporal space as the dictionary and reconstruct the low-dose residue function groups using sparse representation. This sparsity prior is used as a regularization term in the spatio-temporal non-linear processing. In both synthetic data and clinical in-vivo subarachnoid hemorrhage patients, our method outperforms the existing voxel-independent estimation methods.
-
Sparsity-Based Deconvolution Of Low-Dose Brain Perfusion Ct In Subarachnoid Hemorrhage Patients.
[PDF]
Ruogu Fang, Tsuhan Chen, Pina Sanelli.
ISBI, 2012. Oral presentation.
|
|
|
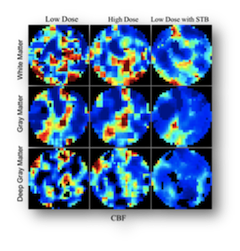 |
Spatio-Temporal Bayesian Method for Noise Reduction in Low-dose CTP
This project is a collaboration with IDEAL lab (Weill Cornell Medical College, New York, NY). A piecewise smooth parametric model with spatio-temporal Bayesian method is proposed to reduce the noise introduced by the low-dose radiation in CT perfusion. On a clinical dataset of 22 patients, the method yields an increase in signal-to- noise-ratio of 78% and a decrease in mean-square-error of 40% at low dose radiation of 43mA.
-
Radiation dose reduction in computed tomography perfusion using spatial-temporal Bayesian methods.
[PDF]
Ruogu Fang, Ashish Raj, Tsuhan Chen, Pina C. Sanelli.
SPIE in Proceedings of SPIE Medical Imaging, 2012.
|
|
|
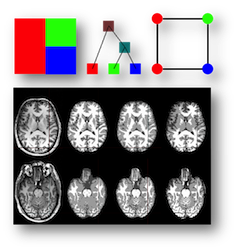 |
Segmentation: Tree-Metrics Graph-Cuts for Medical Image Segmentation
To efficiently segment the medical images into different tissue classes or normal/abnormal regions, we design a tree-metrics graph-cut algorithm that accelerate the graph-cut segmentation. The algorithm composes of three steps: tree generation, tree-metric sweep and tree cutting. The method has been successfully applied to brain tissue segmentation and in-vivo liver tumor segmentation. Improved qualitative and quantitative results were achieved.
-
Segmentation of Liver Tumor Using Efficient Global Optimal Tree Metrics Graph Cuts.
[PDF]
Ruogu Fang, Ramin Zabih, Ashish Raj, Tsuhan Chen.
MICCAI, Workshop on Abdominal Imaging, 2010. Oral presentation.
-
Tree-Metrics Graph Cuts For Brain MRI Segmentation With Tree Cutting.
[PDF]
Ruogu Fang, Joyce Yu-hsin Chen, Ramin Zabih, Tsuhan Chen.
WNYIPW'10. Oral presentation.
|
|
Social Relationship Analysis
|
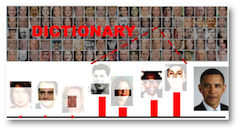 |
Kinship Classification: Modeling Facial Feature Heredity
Understanding how the facial features are inherited and mutated from the parents is a new scientific challenge. To analyze the kinship (parent-child relationship) automatically, we have developed real-time and large-scale kinship classification algorithm, and use it to classify the most likely family that a given unknown person has blood bond.
-
Kinship Classification by Modeling Facial Feature Heredity.
[PDF]
[Project]
[Database (51.8MB)]
Ruogu Fang, Andrew C. Gallagher, Tsuhan Chen, Alexander Loiu.
ICIP, 2013.
|
|
|
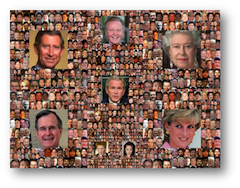 |
Kinship Verification: Automated Kinship Verification from Facial Images
We propose the first algorithm and database for kinship verification problem. We tackle the challenge of kinship verification: using novel feature extraction and selection methods to automatically classifying pairs of face images as "related" or "unrelated" in terms of kinship. First, we conducted a controlled online search to collect frontal face images of 150 pairs of public figures and celebrities, along with images of their parents or children. Next, we propose and evaluate a set of low-level image features that for use in this classification problem. After selecting the most discriminative inherited facial features, we demonstrate a classification accuracy of 70.67%on a test set of image pairs using K-Nearest-Neighbors. Finally, we present an evaluation of human performance on this problem.
-
Towards Computational Models of Kinship Verification.
[PDF]
[Project]
[Database(2.9MB)]
[Demo]
<Ruogu Fang, Kevin D. Tang, Noah Snavely, Tsuhan Chen.
ICIP, 2010. ICIP 2010 Best Paper Award [Link]
|